As the world increasingly becomes data-centric, project managers must harness the power of data to make informed, objective, and efficient decisions. This article will explore how data translates into actionable insights, enhances project outcomes, and drives business success. Whether you're an experienced project manager or a novice in the field, we will equip you with the knowledge to navigate and leverage the dynamic landscape of data-driven decision-making (DDDM).
What Is Data-Driven Decision-Making in Project Management?
Data-driven decision-making is the approach that ensures that decisions are backed by evidence and factual information, reducing the risk of errors and enhancing project efficiency.
Data-driven decision-making focuses on data rather than observation or intuition alone. It involves collecting data from various sources, processing it, analyzing it, and using it to make strategic decisions.
By leveraging data, project managers can gain valuable insights, predict future trends, and make informed choices that contribute to the successful execution and completion of projects.
Data-Driven Decision-Making Process
The data-based decision-making process in project management involves key steps - data collection, analysis, visualization, and data interpretation.
Data Collection
This is the first and most crucial step. Here, relevant data is gathered from various sources like project tracking tools, customer feedback, market research, and more. It's vital to ensure the data collected is accurate, reliable, and relevant to the project's objectives.
Data Analysis
Once the data is collected, cleaned, organized, and analyzed using statistical tools and techniques. This step helps in identifying patterns, trends, and correlations. Data analysis aims to make sense of the collected data and extract valuable insights.
Data Visualization
Data visualization involves representing the analyzed data in a visual format like graphs, charts, or dashboards. Visualization makes it easier to understand complex data patterns and trends. It provides a clear data picture, helping project managers make the best decisions.
Interpretation
The final step is to interpret the visualized data. Project managers need to understand what the data indicates to them about their project's performance, potential risks, and areas for improvement. This step enables project managers to make strategic decisions based on data, leading to more successful and efficient project outcomes.
Data-Driven Strategies in Project Management
- Data-Driven KPIs: Based on factual data, Key Performance Indicators (KPIs) help managers track progress toward project goals. They provide a quantifiable performance measure, helping managers identify improvement areas.
- Data-Centricity: This approach treats data as a crucial asset in project management. It encourages collecting, analyzing, and interpreting relevant data to guide project activities, thus reducing reliance on intuition and experience alone.
- Continuous Feedback Loops: This is a cyclical process of collecting, analyzing, and using data to make decisions. The outcomes of these decisions are then measured and fed back into the loop to inform subsequent decisions, ensuring continuous improvement.
- Analytic Hierarchy Process (AHP): AHP allows for systematic analysis by breaking down decisions into a hierarchy of criteria and alternatives. This helps in making complex decisions more manageable and structured.
- Quantitative Risk Assessment (QRA): It involves using statistical techniques to quantify and predict risks in a project. QRA helps in preparing for potential issues and mitigating them effectively.
- Data-Driven Budgeting: This uses data to make decisions about the budget, providing a more accurate and realistic budget that reflects the project's needs and constraints.
- Real-Time Progress Tracking: Leveraging technology and data analytics, this strategy provides instant insights into the project's progress, enabling managers to make informed decisions quickly.
- Objective Performance Metrics: These metrics, derived from factual data, assess and guide project activities. They ensure alignment with project goals and stakeholder expectations.
- Data-Driven Stakeholder Communication: It includes using empirical data, analytics, and insights to communicate with project stakeholders, ensuring clear, factual, and transparent communication.
- Predictive Project Forecasting: It uses data and statistical techniques to predict future project outcomes. Predictive project forecasting aids in proactive decision-making and effective project planning.
Data-driven Decision-Making Example
The project manager starts by collecting data from various sources, such as previous projects, team capacity, and task complexity. They then use a project management tool such as ActiveCollab to analyze this data, identifying trends, bottlenecks, and areas of inefficiency.
- For example, the data might reveal that specific tasks consistently take longer than expected. The project manager can then make data-driven decisions to address this issue, such as allocating more resources to these tasks, providing additional training to the team, or revising the project timeline.
- The project manager also uses data to track the project's progress in real-time. They set up objective performance metrics, such as task completion rate and bug count, and monitor them closely. If the data shows that the project is not meeting these metrics, the project manager can take corrective action promptly.
- Additionally, the project manager uses data to communicate with stakeholders. They create data visualizations that clearly show the project's status and use these visualizations to keep stakeholders informed and manage their expectations.
- Finally, the project manager uses data for predictive forecasting. They analyze past data to predict future trends, such as potential risks and project completion time. This allows them to plan and make proactive decisions.
Benefits of Data-Driven Decision-Making
Data-driven decision-making improves the accuracy of decisions, enhances predictability, leads to cost savings, saves time, evaluates performance objectively, enhances stakeholder confidence, helps mitigate risks, and drives continuous improvement.
- Improved Decision Accuracy: Data-driven analysis eliminates guesswork, leading to more accurate and informed decisions based on real, quantifiable data.
- Enhanced Predictability: Project managers can predict trends and outcomes with data, improving planning and foresight.
- Cost Savings: Data helps identify inefficiencies and waste, leading to significant cost savings.
- Time Efficiency: Real-time data enables quicker decision-making, saving valuable project time.
- Objective Performance Evaluation: Data provides an unbiased performance measure, facilitating fair evaluations.
- Enhanced Stakeholder Confidence: Transparent, data-based decisions increase stakeholder trust and confidence.
- Risk Mitigation: Data-inspired decision-making helps identify risks early, allowing for proactive risk mitigation strategies.
- Continuous Improvement: Data analysis drives continuous learning and improvement, enhancing project execution.
Challenges in Data-Driven Approach to Decision-Making
Despite the many benefits of adopting a data-driven approach in project management, it comes with challenges. Here are some key hurdles that organizations may face:
- Data Quality: The accuracy and reliability of science-based decisions depend on data quality. Only accurate, complete, and updated data can lead to correct conclusions and good decision-making.
- Data Overload: With the proliferation of data, it can be overwhelming to identify what is relevant and what is not. Extracting meaningful insights from a sea of data can be a daunting task.
- Lack of Skilled Personnel: Data-driven processing requires personnel with the right skills to collect, analyze, and interpret data. There is a significant shortage of such skilled professionals.
- Resistance to Change: Many organizations have a long-standing culture of decision-making based on intuition or experience. Shifting to data-based decision-making can cause team members to refuse this approach.
- Privacy and Security Concerns: With the collection and use of data comes the need to ensure privacy and security. Failing to do so can lead to legal consequences and damage the organization's reputation.
- Integration Challenges: Many organizations have disparate data sources. Integrating these sources to have a unified data view for decision-making can be challenging.
- Cost: Implementing a data-driven approach can be costly. It requires investment in technology, personnel, and training.
- Time: It takes time to collect, analyze, and interpret data. This can delay decision-making, especially when quick decisions are needed.
Project management software that supports data-driven decision-making
ActiveCollab is a project management software that supports data-driven analysis. It provides a platform where teams can easily collaborate, organize tasks, and track project progress. This software offers various features such as task management, time tracking, invoicing, project budgeting, and reporting.
One of the remarkable ways ActiveCollab supports science-based decision-making is through its comprehensive reporting feature. Users can generate detailed reports, which provide insights into project performance, efficiency, and profitability. These reports can be customized to suit the needs of the team or the project, allowing for more precise analysis and decision-making.
Additionally, ActiveCollab's time-tracking feature is a valuable tool for DDDM. It allows managers to track the time spent on each task, enabling them to identify inefficiencies and make adjustments where necessary.
ActiveCollab's project budgeting feature also provides real-time information about the financial status of projects. This data can be used to make informed decisions about resource allocation, cost management, and overall project financial health.
ActiveCollab's various features support data-driven decision-making by providing valuable insights about project performance, team efficiency, and financial status. By leveraging these data, managers and teams can make informed decisions that drive project success.
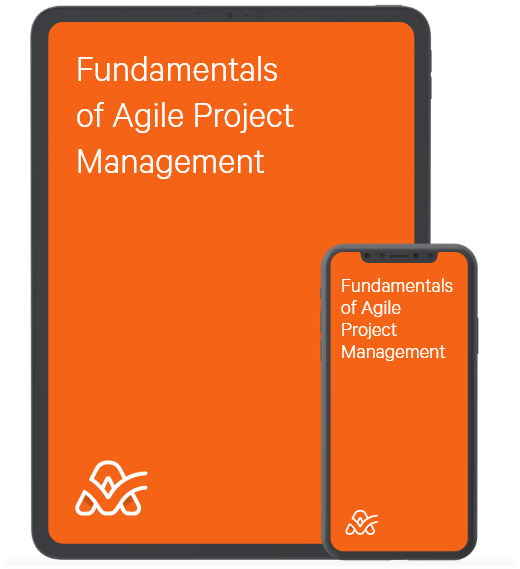